Algorithms for efficient analysis of single cell data
Robust and precise characterisation of cellular phenotypes
Our recently developed ability to peep into molecular portraits of individual cells provides an exceptional resolution for viewing the emergence, dynamics, and fate of cellular phenotypes. However to extract all this, one has to tackle the large dimensions and noisy readings associated with the single-cell omics readouts. Our lab specializes in developing computational methods to tackle these problems. In the past, we developed some of the speediest, resource-friendly, and accurate computational methods for single-cell clustering (dropClust, NAR 2018a), rare cell detection (FiRE, Nat. Comm. 2018), cell atlas searching (CellAtlasSearch, NAR 2018b), differential expression analysis (ROSeq, Genome Res. 2021), etc. These methods fuse elements of statistics, machine learning, and Big Data algorithms. Currently, we are leveraging our experience in handling single-cell transcriptomics data to solve higher-order mysteries, such as non-genetic signatures of competitiveness among cancer clones, and linking RNA stability with cell-signaling dynamics (bioRxiv 2021).
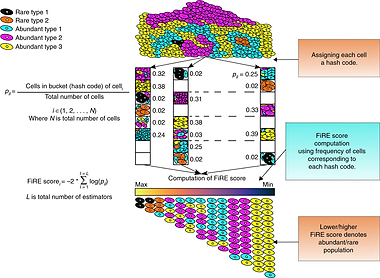

Lone Cancer Cells
Hunting expression based hints to cancer from blood and tracking cancer-immune interaction dynamics
Our obsession with gene expression, landed us into cancer since cancers are gene expression kaleidoscopes and a beautiful system to explore RNA elasticity. In the context of cancer, there are a few unsolved paradigms that interest us the most. One of those is how to recognize all phenotypes of Circulating Tumour Cells that float around in peripheral blood (JCM 2020) (bioRxiv 2021). As we know cancer cells undergo molecular remodeling to successfully traverse distant organs. Only a fraction of such disguising strategies is known to us. One is Epithelial to Mesenchymal Transition or EMT. Current best practice methods are limited to selection for size or canonical markers such as EpCAM. We use a unique combination of unbiased, microfluidic enrichment of CTCs and gene expression pattern recognition to detect CTCs irrespective of any specific marker. Along similar lines, we developed and commercialized the Tumour Educated Platelet (TEP) gene panel for early cancer detection (BMC Genomics 2021) (CareOnco). More recently we interrogated NK-Cancer cell interaction at doublet resolution and learned signatures associated with killing by NKs. Notably, not every NK cell kills every cancer cell that comes in its contact. A different ongoing study alludes to single cell-based signatures that are associated with clonal advantage within a tumor ecosystem. Watch out for future developments.
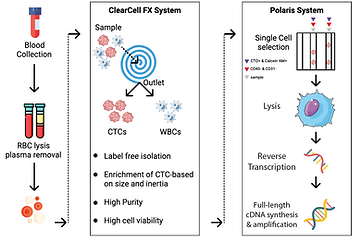

Fun Projects
We do a lot of fun projects without a pointed question
We do many fun projects, which often do not lead to significant pointed findings. However, such projects give us a lot of joy. Here is an example. With a quarter less than a decade's experience in single-cell research, we realized while cell-atlasing for healthy tissues is kind of popular, it may be tough to create single-cell atlases for diseases. While we do not have the arsenal to create such resources, we decided to use Text Mining and Natural Language Processing to scavenge ~18 million PubMed abstracts to create PathoMap that tells us which genes are HOT in which organs in terms of their pathological role. Notably, Pathomap, by the virtue of its underpinning theory, also learns transitive relationships. Pathomap provided a vector representation of words indicating a possible association between DNMT3A/BCOR with CYLD cutaneous syndrome (CCS) (bioRxiv 2022). The first manuscript reporting this finding was not part of our training data. isn't it mind-blowing?
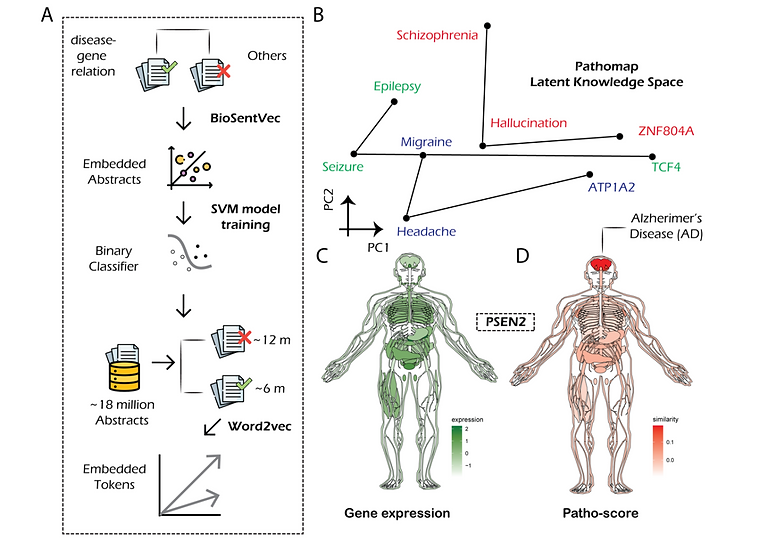
Funding
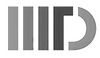_edited.png)
_edited.jpg)
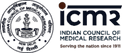_edited.png)

